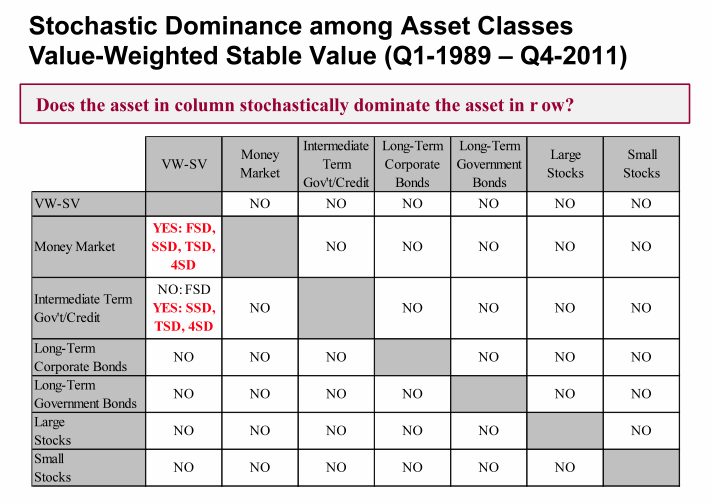
In Part I of our performance review of stable value investment funds (SVIF), we utilized mean-variance analysis in forming optimal asset allocations for six asset classes, including long-term government bonds, long-term corporate bonds, intermediate-term government/credit notes, large stocks, small stocks, and money markets.
We found that when SVIF were added to the mix, the efficient frontier was significantly improved, and that several asset classes were no longer included in any of the portfolios along the efficient frontier. We also used the Sharpe Ratio and the Sortino Ratio to compare the reward-to-risk ratios of the various asset classes, and found that SVIF scored the highest among all asset classes, and by a substantial margin.
Although widely used, the mean-variance framework and the performance ratios associated with it have serious limitations. Strictly speaking, their use is justified under conditions that are not even closely met in the real world.
Over the past 50 years, volumes have been written in the economic and financial literature on modifications to the mean-variance model, either based on different assumed utility shapes or on more realistic assumptions on return distributions, in an effort to address the deficiencies of the approach while retaining some of its attractive attributes.[1]
Stochastic dominance analysis
A powerful technique was developed in the 1970s to remedy the problems of mean-variance approaches. It is known as Stochastic Dominance (SD), and its validity depends on no particular asset return distribution nor any particular utility function.[2]
Stochastic Dominance comes in various degrees. First-degree stochastic dominance (FSD) imposes only one preference restriction—investors prefer more wealth to less wealth. If an asset class exhibits first-degree dominance over another asset class, it should be preferred by all investors, regardless of whether they are risk seekers, risk neutral, or risk averse, in any degree.
If an asset class exhibits second-degree dominance (SSD) over another asset class, it should be preferred by all who are risk-averse, in any degree. However, investors who are risk seekers or risk neutral may or may not prefer it.
Third-degree dominance (TSD) is for investors who prefer positive skewness of asset returns, and fourth-degree (4SD) takes into account the aversion most investors have for extreme return distributions, where positive and negative outcomes are just as likely.
The table below presents the SD results among the seven asset classes in our study, based solely on historical returns. Only the SVIF historical returns distribution dominates two asset classes in the stochastic dominance sense up to the fourth degree; none of the other asset classes dominates SVIF.
However, over the longer time period beginning in 1973, SVIF did not dominate money market investments by the first-degree, yet they did dominate by second-degree and higher. This suggests that for investors with any degree of risk aversion, however slight or strong, SVIF should be preferred to money market investments.
Although SVIF failed to dominate intermediate-term government/credit notes by the first degree, they dominated by the second and higher degrees. This result is a direct consequence of the fact that positive intermediate-term bond returns during the period of our study were never large enough, relative to corresponding SVIF returns, to make at least some risk-averse investors prefer the riskier intermediate-term bond investment. When our time period of analysis was extended to 1973, the same dominance was maintained.
These results are remarkable. Not surprisingly, there is no stochastic dominance of any one traditional class over another; indeed dominance is rarely encountered among asset classes. Accordingly, it was surprising to find that SVIF dominated two of the major traditional investment classes.
Multi-period optimization analysis
Multi-period investment theory was developed decades ago in response to the drawbacks of single-period models such as the CAPM and others. Contributors to the theory and developers of investment models based on it included such luminaries as Paul Samuelson (MIT), Nils Hakansson and Hayne Leland (Berkeley), Steve Ross (Yale) and Gur Huberman (Columbia), among others.
Here we provide only an intuitive sense of its underpinnings and insights. Multi-period investment theory has been dubbed the Turnpike Theorem, and for a good reason. Consider the fastest way to drive from point A to point B, located a few miles distant. Generally, the most direct way along local roads is the quickest if the distance is short (assuming that a turnpike does not already connect the two points).
However, if point B is a long way from point A, say 20 or more miles, it might be faster to travel out of your way to connect to a turnpike or freeway, exit as close as possible to point B, and travel the rest of the way on local roads. This turnpike alternative generally becomes more and more attractive as the distance between point A and point B increases, and is almost certainly the preferred path to follow (if speed of arrival is of primary importance) when the distance exceeds a hundred or so miles.
Similarly, in multi-period investment theory, if one’s investment decisions are guided by a single-period optimization model such as the CAPM, among many others, the resulting wealth accumulation in the long run is very likely to be significantly lower than if one were to follow a dynamic investment model.
Dynamic models based on the theory are designed to maximize the investor’s expected utility of wealth where risk is measured by the degree of utility diminishment incurred from investing in risky assets. The economists referenced above uncovered a particular class of power utility functions that is consistent with maximizing long-term wealth for any level of risk aversion. Portfolios based upon such utility functions are known as turnpike portfolios.
One of the nicest features of such long-term investment models is that they are consistent with myopic behavior. In other words, if you invest this period according to the model, your behavior will be consistent with investing in a way that maximizes long-term wealth. However, if you follow investment models based on maximizing alternative utility of wealth functions or otherwise, you will do worse.
In this review we won’t rehearse the considerable safeguards we underwent in designing and implementing the dynamic model to assess asset performance.[3] Instead, we will simply present some representative results from which asset performance can be evaluated.
We derive the optimal asset allocations for each quarter based on the quarterly returns joint distribution across all asset classes considered from the prior 80 quarters. This length of time is sufficient to incorporate two or more business cycles, providing robust estimates. We use the full joint empirical return distribution of alternative investments in order to determine optimal wealth allocations that depend on the risk aversion parameter of the investor.
All moments of the joint distribution (e.g., mean, variance, skewness, kurtosis, etc.) are taken into account. The expected returns for the next quarter are derived from market data, but in the case of equities, the risk premium is an input reflecting the investor’s expectations. From this joint distribution, we apply the turnpike utility function to derive those asset allocations consistent with long-run maximization of utility of wealth.
Two fairly typical sets of outcomes are presented below. Each optimal asset allocation will depend on the investor’s level of risk aversion, ranging from 1 to 75 (logarithmic, or only slightly averse, to extremely risk intolerant), and across a variety of assumed annual equity risk premia on large stocks ranging from 2.5% to 5.5%.
Consistent with past research, we consider a risk index of 5 to be fairly moderate, although recent research suggests that the average risk aversion in the population is considerably higher, ranging from 20 to 45.
The figure below, for the first quarter of 2010, reveals that, with the exception of a very small proportion of long-term government bonds for moderate risk aversion levels when the large stocks premium is 5.5% per year, only small stocks and SVIF are relevant in the optimal portfolios, irrespective of the level of risk aversion.
As expected, SVIF represent a larger fraction of the optimal portfolio as risk aversion increases. Also as expected, a larger equity premium increases the proportion of small stocks in the optimal portfolio for any given risk aversion level.
Next we show optimal portfolios for the third quarter of 2008, again using data for the 80 quarters that precede it. The figure below shows the optimal portfolio weights for selected values of the risk parameter, and selected large stocks expected annual risk premiums.
Even though expected returns across all asset classes were significantly different from those used in calculating the 2010 optimal weights, we observe roughly similar optimal allocations, with small stocks and SVIF being the only significant investment classes, except for a small fraction of long-term government bonds at higher large stocks premiums.
This pattern of optimal portfolios being comprised largely of SV and small stocks is not that uncommon, it turns out, when expected returns are based on historic spreads and current yields. In fact, in our three separate studies, we found these portfolios to be the norm, but with interesting occasional exceptions.[4]
The analyses we have conducted to date conclude that, for moderately and highly risk-averse investors, SVIF are, under reasonable yield curve assumptions, a major component of an optimal portfolio, to the exclusion of money market funds and the near exclusion of intermediate-term bonds.
Since their inception in 1973, SV funds have undergone several severe tests. They survived the market fallout from the OPEC cartels, the severe stock market declines of 1973-4, 2000-2002, the interest rate spikes of the early 80’s, the stock market crash of 1987, the liquidity and credit spread crisis of the late 90s, the plummeting interest rates of the 21st century, economic booms and deep recessions, the terrorist challenges to financial markets in 2001, and countless other daunting circumstances. With very few exceptions, they appear to have weathered the recent financial crisis of 2007-8.
Concluding remarks
In Part I and Part II of our brief review, we found that despite the different focus of the three performance evaluation methodologies used, the results we obtained under each analysis reinforced each other in the sense that SVIF outperformed some of the alternative investments we considered across one or more dimensions.
Whether SFIV will be appropriate for your clients will depend on their levels of risk aversion, liquidity needs, and other elements of their asset portfolios. If a determination is made that SVIF could be a valuable element of their portfolio, an assessment of providers will need to be made.
David F. Babbel, professor emeritus, The Wharton School, Univ. of Pennsylvania, is a Senior Advisor to CRA (Charles River Associates) and leader of its Insurance Economics practice. Miguel A. Herce, Ph.D., is a Principal at Charles River Associates.
© 2013 RIJ Publishing LLC. All rights reserved.
[1] Either of two strong assumptions can be used to justify reliance on the mean-variance approaches, and the CAPM in particular, to portfolio composition. If investor preferences (utility) can be accurately mapped by a quadratic function (which has a shape similar to the cross section of a dome, indicating that more wealth is preferred to less, but at a diminishing rate as wealth increases, and beyond some point of wealth attainment, less wealth is preferred to more wealth at an increasing rate of repugnance for wealth), or if asset returns can be adequately characterized by a Normal distribution, then the basic mean-variance models including CAPM are supported.
[2] The economic foundations and mathematical support for the stochastic dominance criteria and implementation are given by Haim Levy, Stochastic Dominance: Investment Decision Making under Uncertainty, 2nd Edition, Springer, 2010.
[3] Our previous studies were published in the Working Papers Series of the Wharton Financial Institutions Center website (Babbel and Herce, 2007-#21, 2009-#25, 2011-#1). http://fic.wharton.upenn.edu/fic/papers.html The 2011 study features our most advanced implementation of the dynamic investment model.
[4] A thorough review is in Babbel and Herce, 2011, at: http://fic.wharton.upenn.edu/fic/papers/11/p1101.htm