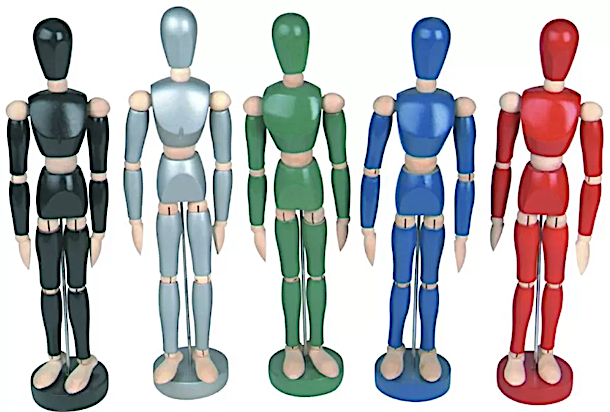
Life insurance companies are using predictive analysis, derived from substantial insurer historical data, as the basis for modeling variable annuity owner behavior (OB). An important metric for predicting OB is the degree to which a contract is “in the money” (ITM).
The insurer is concerned that when the liability (present value of the future estimated owner income) is substantially greater than the account value, the owner will elect the guaranteed income and the insurer would then be on the hook for the estimated difference. But how can contract owners or their advisers know when the contract is ITM, so that they could take advantage of this situation?
The CEO of an actuarial firm that predicts OB recently told Retirement Income Journal:
Some issuers argue that annuity owners are not actuaries or calculating machines. So, they’ll look to simpler proxies, like ‘nominal moneyness.’ Others believe that while annuity owners aren’t doing detailed calculations, they have some sense of actuarial/economic value. So, they use an actuarial moneyness measure. We spend more time talking with our clients about this issue than anything else, because it is critically important to the long-term profitability of these products.
Can owners “intuit” a contract’s ITM? As an actuary, I’m skeptical. Most VA contracts have many moving parts; Estimating the ITM would require both an excellent method and a substantial programming effort. But, as shown below, these kinds of apps are fast becoming a reality. Spurred by growing owner awareness, and recent Best Interest regulatory requirements, they’ll likely have a major impact on OB.
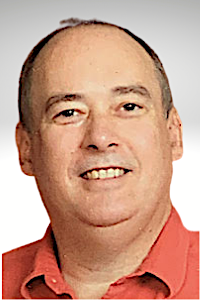
R. Michael Markham
However, the CEO’s comment above raises a useful and important distinction. This actuarial/economic value is an insurer’s liability, based on their finances; whereas, for the owner, the value would be an asset, based on their finances. These two values must be different, and so must their ITMs.
“Nominal ITM” would be a crude approximation, but it does establish the principle of a separate owner-centric ITM, one that the owner could use to optimize their income. The issuers would need to modify their OB models to account for the owners/advisers’ reactions to this or similar metrics.
So, what are the challenges in developing a more useful insurer’s OB model?
The need to jump the insurer/owner divide
To develop an OB model, insurers would first need to jump the insurer/owner divide. That is, put themselves in the shoes of the owner/adviser, and ask how, at any point in time, they would perceive the available options. Then they’d have to try to predict which current and future choices would maximize their ITM-ness.
The overarching principle must be that the calculations are based on the owners’ perspectives and on their attributes, not the issuers’. The owners see the world from their own financial perspectives. They’re probably not aware of how the insurer manages its finances. They care only that the policy fulfills the guarantees in the contract.
How the ITM is calculated
What are the actual (and optimal) mechanics for calculating ITM? If we assume that each future year-end has its own ITM, which one(s) would be utilized? Further, would the insurer’s actuarial ITM (I-ITM), or nominal ITM (N–ITM) determine OB, or some weighted combination of the two?
The general equation for ITM is:
ITM = (PV Future Income – Account Value)/Account Value
This equation looks simple. Far from it.
- There are many possible future scenarios, each with numerous possible income streams.
- There are several ways that the PV component could be calculated; from deterministic to stochastic.
- There are many approaches for determining the benefits and how they would be discounted.
Here’s a diagram showing some possible income streams, which could also include different combinations, such as a stream of free withdrawals, followed by the election of a guaranteed income stream, followed by, in some cases, when allowed, a death benefit or a surrender.
If owners are “not actuaries or calculating machines,” how could they determine any ITMs for these complicated variable products?
How to address this challenge
There are two kinds of ITMs: the actuarial one, observed by the insurer (I-ITM), and the one observed by the owner/adviser (O-ITM). This distinction is critical. Owners will decide based on the O-ITM, not the I-ITM.
The owner will rely on an ITM that is based on the contract’s relevant details, of course, but equally on the owner’s age, gender, risk-appetite, estimated longevity, benefit preferences, tax brackets, targets, legacy, and other goals. The O-ITM is a function of all these things.
Ask the question: what lump sum would the owner need to invest today in a no-load mutual fund portfolio, based on their risk-appetite, to self-fund the contract’s projected owner net income stream? The answer can be computed by simulating their investing in a mutual fund whose portfolio matches their risk-appetite, and by deducting the contract’s periodic income payments, with the last deduction resulting in a zero balance.
The lump sum is what their O-ITM asset would be worth to them today. This asset would then be the PV component of the numerator used in the O-ITM calculation. Discount rates used for contract income would equal the net returns of this owner-centric portfolio. In fact, this is critical for what comes next: portfolio returns make sense only in a specific economic scenario.
‘Most Frequent Gambit’
Obviously the future is mostly unknown. There are many, many possible future economic scenarios. To address this challenge, the best approach is stochastic, i.e., projecting O-ITM distributions derived from numerous calibrated, randomly generated economic scenarios.
These economic scenarios would be combined with current user-entered policyholder attributes, to help match present and future decisions based on owner goals.
The metric for evaluation is the PV of net income, and we assume that the owner aims to maximize this figure. This is a common theme in mathematical approaches. But this “goal seeking” needs to be developed and applied in a way that the adviser and owner will understand and accept.
Broadly considered, we can begin to locate the maximum by considering every possible “start year/benefit type” gambit. In each scenario, each such “gambit” produces its own O-ITM figure. But we need to sift the resulting figures to estimate the owner’s most likely choice (OB). This determines the contract’s overall O-ITM.
So, what are the steps for calculating the OB, and the contract’s overall O-ITM?
- Project numerous economic scenarios and determine benefits for the targeted range of years.
- For each scenario/targeted year, produce an O-ITM frequency distribution for the maximum gambits for each scenario.
- Using this distribution, identify the most frequent gambit (MFG).
- For each targeted year, in each scenario, use the MFG to determine the O-ITM for each year and record each year’s gambit.
- Starting at the evaluation year, check each scenario, and, if its MFG occurs in that year, then that gambit will be used to calculate the scenario’s unique and final O-ITM, as of the evaluation year.
Note that all income streams are discounted based on the scenario’s returns, for a shadow no-load mutual fund portfolio based on the owner’s risk-appetite.
Also note, each year’s frequency distribution is derived from the assumption that the owner is running the O-ITM app at that time, within each scenario. Due to the very large number of calculations required to do this, for each scenario and each projected target year, approximate methods will be needed.
B-I requirements spur online apps
The app described so far seems theoretical, but the demand for O-ITM apps is not. Advisers, broker-dealers, and producers will be stepping up their game for their clients when selling, replacing, or managing variable annuity contracts. They will need to comply with new regulations that address new fiduciary responsibilities, such as the SEC’s Reg-BI, new standards from both the CFP Board and the Department of Labor and multiple states’ Best Interest requirements (e.g., New York’s Reg 187 or Arizona’s SB1557).
Several watchdogs will be looking over their shoulders; advisers will need to evaluate contracts before and after purchase, providing on-going due diligence and account-management advice. The Biden administration is likely to enforce Best Interest and strengthen the fiduciary requirements for anyone selling or managing these kinds of products. New online apps, such as SmartAsset, are help potential policyholders pick the most reliable advisers. Every adviser will need to bolster a fiduciary relationship with clients.
That’s exactly what’s happening. A method called BI-SEM, from the BI-SEM group, has been proposed. It addresses the need for a standard stochastic approach to the evaluation of variable deferred annuities and deferred annuities in general. Second, apps are now emerging to implement the method.
BI-SEM (Best Interest – Stochastic Evaluation Method)
BI-SEM is a new stochastic evaluation method that bases its O-ITM on a policyholder’s key attributes, and discounts future estimated net income based on the policyholder’s risk-appetite (see BI-SEM whitepaper). I believe the BI-SEM method, when implemented correctly, provides an excellent O-ITM model for owners/advisers.
As a stochastic method, BI-SEM employs important features from the NAIC’s Commissioners Annuity Reserve Valuation Method (CARVM) and Principles Based Reserves (PBR). In their whitepaper, the group emphasizes piggybacking on existing, well-vetted statutory valuation procedures. Any app that properly employs this method should significantly impact OB.
In addition to Age and Sex, the Method makes use of the following valuable attributes in its projections:
- Risk Appetite
- Health Status/Estimated Longevity
- Goals and Strategies
- Tax Brackets
- Benefit Preferences
- Retirement Age Targets
- Legacy Wishes
In most cases these are attributes not known to the Insurer. BI-SEM emphasizes that, unlike the Insurer, an adviser can ask these personal questions. In fact, for Best Interest, they must.
When implemented properly, the method is a sound one for the owner’s interests, because it:
- Takes advantage of important personal attributes known only to the policyholder.
- Is generally based on current well-vetted US Statutory Valuation Methods.
- Is stochastic – a better simulation of real life from more viewpoints.
- Calculates a current dollar evaluation of a contract.
- Calculates a Score for the contract
- Provides dispersion statistics.
- Uses standardized metrics for contract comparisons.
A BI-SEM App from InjAnnuity, Inc.
This discussion is not purely theoretical. O-ITM apps already exist, on the internet. The DARMA online app authored by James Kavanagh, a founder at InjAnnuity Inc., is an excellent implementation of BI-SEM. The screen shots below highlight its versatility.
The app produced meaningful reports and charts and did an excellent job of proving its numbers with a comprehensive set of on-demand audit reports (see below).
The existence of these kinds of apps, and their easy accessibility on the internet, greatly strengthens the case for the O-ITM-centric OB model.
Getting it right
Usage of O-ITM-based apps is not widespread—yet. The approach now needed by insurers is to assume an OB Model where the owner/adviser is much more informed about O-ITM. They need to assume that they are using a sophisticated app to best the insurer.
The overarching need in the model is to base owner/adviser decision-making from their now better-informed owner-POV. For the best results, the model should be stochastic. The big challenge will be in estimating owner attributes that are not known to the insurer. They can be based on the information contained in the contract. For example, an owner’s risk-appetite might be derived from their subaccount portfolio.
Other attributes might be derived from elected riders. Targeted retirement ages and other owner goals could be derived from historical experience for policyholders in the same cohorts. This would be combined with attribute probabilities derived from the historical studies performed by suppliers like Ruark, LLC.
OB assumptions should now be updated to consider that a certain percentage of policyholders are O-ITM aware, N-ITM aware and recognize that this is a moving target as O-ITM-aware apps proliferate. Getting this right is critically important to the long-term profitability of these products.
© 2021 R. Michael Markham.